Authors: Oliver Lukitsch, Thomas Fundneider
We have been witnessing a major shift in our learning landscapes, especially in higher education. The importance of the generalist has declined and that of experts has increased. We are faced with exponential knowledge growth, and the accumulated knowledge is more accessible to us than ever before, through digitization.
You could also say we are in the age of ubiquitously accessible knowledge. This already has and will likely have greater significance in the future, shaping our ways of teaching and learning in our campuses.
The core of this development is the emerging possibility that we no longer have to “internalize” knowledge, i.e. learn it by heart. It seems too arbitrary given the amount of knowledge available. And it seems all the more important to train the ability to assess and use the knowledge and available information critically for decision making and reflection.
Perhaps because there has been an agreement on the importance of this all along, today’s focus is on what digital tools can do to provide us with information quickly and smoothly. The focus is primarily on smart, digital knowledge delivery. Given that knowledge workers need to quickly consult an ever-increasing knowledge base on a daily basis, this focus also seems commonsensical.
The digitization of our knowledge economy also means that our visions of the future of our educational landscapes are almost visions of virtual spaces. According to this vision, physical space will continue to be needed, but it will be degraded to a basal infrastructure that by itself does not fulfill any primary educational function. The age of ubiquitously accessible knowledge seems to be pushing physical space into the background.
Cyber-physical educational spaces tend to treat knowledge as an object. However, the most basic form of knowledge is embodied.
In this blog post, we want to show that we must not only critically observe this development but also highlight the essential importance of the physical, built environment for educational spaces. Our point is this: smart knowledge technologies will lead to those human competencies becoming more important that cannot be outsourced to the digital domain. But it is precisely these competencies that require the physical, built learning environment to be developed positively.
Our blog post is about understanding why this is the case. In short, cyber-physical educational spaces tend to treat knowledge as an object (i.e. an object just like the physical symbols we use to encode meaning). However, the most basic form of knowledge is embodied. It is brought about and enacted by human activity, and therefore cannot be treated as an object that can be stored and transmitted across cyberspace. Rather, human knowledge is profoundly embedded in and afforded by the physical environment. But before we get into that in more detail, we must first introduce the protagonist of our post.
Bound in the Ether: Knowledge has left the building
It is one of the most important features of our future learning landscapes: we do not only administer an exponentially growing set of knowledge. We access it more seamlessly and quickly than ever before. The knowledge of human kind is “ubiquitously available” (Friedman et al., 2016).
At least in principle, what we want to know is just a few clicks away. We can “google” it or we can search Wikipedia – and for academic purposes, we can search scientific databases on a conventional browser, just to mention the most prominent means of knowledge extraction. That is not to say that we are able to interpret or use all this knowledge. But as long as we know what we’re looking for, we can find it.
A synergistic driver of this infrastructure is, of course, the exponentially growing amount of knowledge. Without digital infrastructure, we can no longer process such volumes of knowledge in a timely manner, and this applies to students and, above all, to researchers and practicing scientists.
Moreover, it seems increasingly arbitrary to decide what bits of knowledge we should “memorize”. The Socratic paradox (“I know that I know nothing”) becomes more pertinent than ever before. Only today, it sounds less like a wise admission of ignorance.
The ubiquity of knowledge is not a surprising feature of modern-day knowledge work. It is taken for granted by teachers, researchers, and students alike. However, as of today, the impact of this development has not yet profoundly changed the way we learn or teach. One reason for this is that today we are dealing with more or less the same kind of interfaces that we already know from the pre-digital era. In fact, the most relevant interface for (academic) education is an almost unchanged entity.
Since the birth of universities, the piece of writing, the paper, the book has been thought of as the interface for the transmission of knowledge. The digital tools we have now are nothing more than an extension of the original. In principle, it doesn’t matter at all whether we learn in a library or on a computer. We read symbols on paper or on the screen.
Now, however, it is precisely those interfaces that will be increasingly called into question in the future. The advent of smart technologies (i.e., automated systems) may fundamentally change our learning experience at universities. They could fundamentally revolutionize the way we deal with the ubiquitous availability of knowledge.
This takes us to the vision of the knowledge cloud.
Learn to Learn: How to engage with the knowledge cloud
The more our knowledge bases will grow, the faster and smoother, but also more intelligent, the retrieval of this knowledge should be. Friedman et al. (2016) thus conceived of a (hypothetical) “knowledge cloud”, a smart cloud storage that contains all the knowledge of a given domain, for instance, a biomedical cloud. Upon request, such a cloud can provide any kind of information in written or spoken form.
At first sight, it resembles Wikipedia – with a slightly more sophisticated interface. However, the vision of the knowledge cloud is more radical. Knowledge in “computable form” (ibid.) can be used to answer the user’s question on the fly. For instance, a physician might want get advice for her treatment of a patient, providing the cloud with contextual information while the cloud suggests a way forward.
Subscribe to
our newsletter
Interested in topics, such as the future of learning? Subscribe to our monthly newsletter to read more articles like this.
Therefore, the knowledge cloud fundamentally shifts the way we deal with knowledge. As the cloud is always in our vicinity, we can consult anytime, expecting reliable results. Of course, the vision is not to consult the cloud for the purpose of memorizing knowledge long term.
Friedman and colleagues (2016) stress that what matters is the ability to know what one doesn’t know, to ask the right questions, and to make decisions when the cloud provides conflicting information (and thus under uncertainty). Hence, the educational focus of the future will be to train students to deal with external knowledge, rather than to internalize (i.e. to memorize) it.
However, the emergence of the knowledge cloud will also coincide with a major revolution in how we use digital interfaces. Friedman et al. (2016) think of their knowledge cloud as accessible via our smartphones. But our smartphones are only one point of contact in the growing “internet of things” (IoT). And indeed, future learning environments are expected to be embedded in the IoT.
Smart Learning Environments
This takes us to another development of future learning spaces – one that will affect (and complicate) how we will engage with the knowledge cloud. The future of learning spaces is often conceived of as “smart learning environments” (or SLEs, for short). There are different definitions for what such smart learning spaces are. Yet, they all have in common that they involve smart sensors and “actuators” (devices that act on their own).
Such smart, automatized systems adapt to the learning needs of their users, such as monitoring their progress and providing according information. They show students their weaknesses and how they perform with respect to others and they provide them with the digital tools to deal with their shortcomings. While some definitions emphasize technology, others put the human center stage. Accordingly, SLEs should foster reflection, innovation, conversation, and self-organization (Gros, 2016). Considering this, smart learning environments tend to be so-called self-regulated learning environments (SRLs). Their smart design often fosters “goalsetting, helping-seeking, time management, and self-evaluation” (Gambo & Shakir, 2021).
Considering this, what do SLEs mean for the ubiquity of knowledge and thus the knowledge cloud?
Library 4.0
Noh (2015) provided us with an early vision of the knowledge cloud as being embedded in WEB 4.0. He imagines a digital library as a place of symbotic human-machine interaction: the library 4.0.
He defined it as “intelligence-based, massive data, augmented reality, context aware, cutting-edge displays, and infinite creative space” (p. 786). Today, this still sounds rather visionary. Yet, considering the technologies we have, it’s just a matter of time and investment to make it happen.
Yet, considering all this, one thing remains noticeable. Several accounts of smart learning environments have in common that they do not provide any clear guidelines for what this means for the built environment (Freigang et al., 2018).
The Digital-Physical Divide: The decline of physical space?
Even though future learning landscapes are conceived as cyber-physical spaces, and even though interfaces become increasingly “transparent”, one can witness an odd negligence of physical space. What is (or will be) neglected (by designers of and experts on learning spaces) is precisely how physical space can accommodate the needs of students embedded in a knowledge cloud – in a virtual knowledge ecosystem.
Today, we pay attention to smart technologies but the physical places in which they manifest remain a void (yet to be filled with meaning). This can have a variety of implications. It opens up new degrees of freedom for designers to create physical spaces that are not constrained by physical artifacts such as those found in large numbers in a library. Such spaces only need to accommodate increasingly smaller smart devices – other than that, they are not constrained in any way. While this gives the designer more freedom, it also means devaluing the physical space as a genuine physical learning space. It seems that when considering the demands of learning environments, the physical space will be “unburdened” or relieved. The virtual space takes over many of its tasks completely. (Just think of the digital classroom, which has gained importance in the wake of the pandemic).
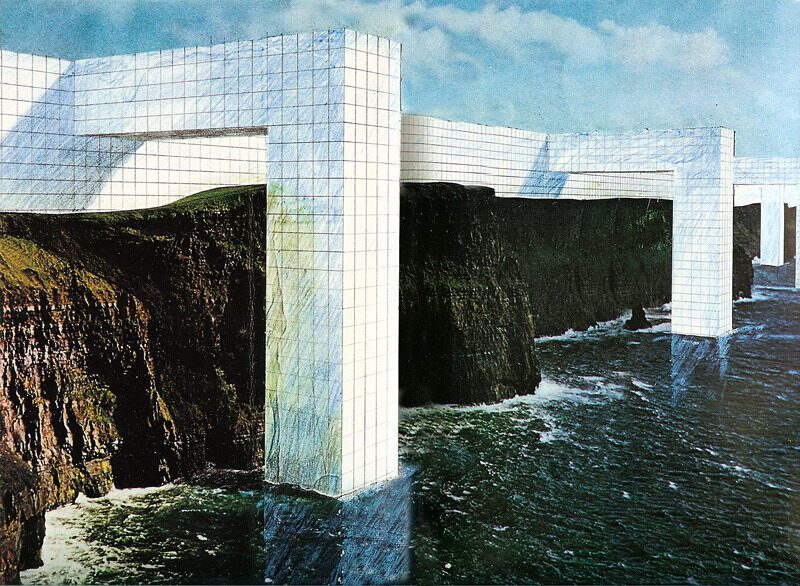
The upshot: talk of smart interfaces in cyber-physical learning environments suggests that we have a holistic solution on hand. It seems that such cyber-physical places will be highly integrative and that within them, the boundaries between physical and digital space are merged.
Yet, we are far from such a degree of envisaging or understanding such “intimacy” (Haugeland, 1993) between the digital and physical space. The boundaries between the physical and digital world stand firm. As it were, physical and digital domains stand side by side giving each other space.
The reason for this is that, as of now, we seem to lack design principles that can merge digital-physical spaces in a purposeful way. Such principles should guide us through designing physical spaces that can accommodate virtual spaces and vice versa.
Here, we stand at a crucial crossroads. We might design physical spaces based on how they can accommodate digital devices for “smart learning”. However, such an approach has not produced any physical design principles to date. Rather, it has led to the designer of physical places being granted fool’s license (“Narrenfreiheit”).
We propose an approach to this that is as obvious as it is human. It is not yet an answer to what the physical component of future educational landscapes should look like. Our approach is only intended to show how to think about educational spaces so as not to decouple their design from emerging technologies.
Our approach is simply to take seriously the most basic types of human learning. As we have seen so far, smart knowledge technologies will render specific human (learning) competencies more important that cannot be outsourced to the digital realm. And it is exactly these competencies that require positive development of the physical, built learning environment.
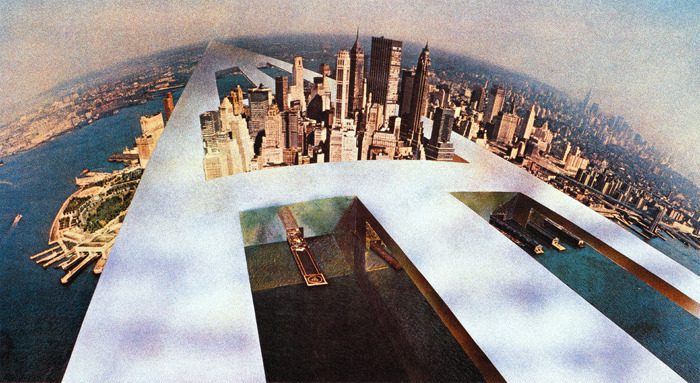
Four Types of Learning
The way we use artifacts (such as smart tools) for the purpose of learning is highly dependent on the way we learn. To understand how, let us take a closer look at the different modalities of learning. Moreover, since the knowledge cloud (and the ubiquity of knowledge) has been a centerpiece of this blog post, we will consider how our ways of learning affect the way we engage with the knowledge cloud.
Linear Learning
Linear learning is what we do when we memorize the medical term of a specific muscle or the date of a historic event. In the past, students consulted their textbooks with the ideal result of memorizing the target information. Consulting the knowledge cloud for the purpose of linear learning, then, is a highly simplified, frictionless version of looking up information in a book. Only by doing it with the aid of the knowledge cloud, searching for information is a highly convenient and smart process.
Considering the ubiquitous availability of knowledge, linear learning is losing significance (as a human skill). There is too much knowledge to be internalized so that it becomes increasingly reasonable to offload facts into the cloud and access them whenever needed.
The level of linear learning is where the vision of a knowledge cloud is so forceful. The vision is that linear learning can be unburdened by requiring us to remember less. For the cognitive scientist Andy Clark, this would turn us into cyborgs whose factual knowledge is outsourced to a machine.
More importantly, the knowledge cloud does not only relieve linear learning systems. It also increases the importance of other forms of learning.
However, while the knowledge cloud can support (and even replace) linear learning, no argument has been made of how it could possibly support other, perhaps more fundamental forms of learning. The reason for this is that the forms of learning described below are highly embodied and emphasize physical actions and interactions in physical space.
Another way of putting this is that linear learning treats knowledge as data, stored in physical symbols (i.e. the symbols stored on our digital devices). It treats knowledge as an object. However, human knowledge is much more extensive than this and must be understood, in its most primal form, as an embodied process or activity. Knowledge is actively brought about or enacted.
Single Loop Learning
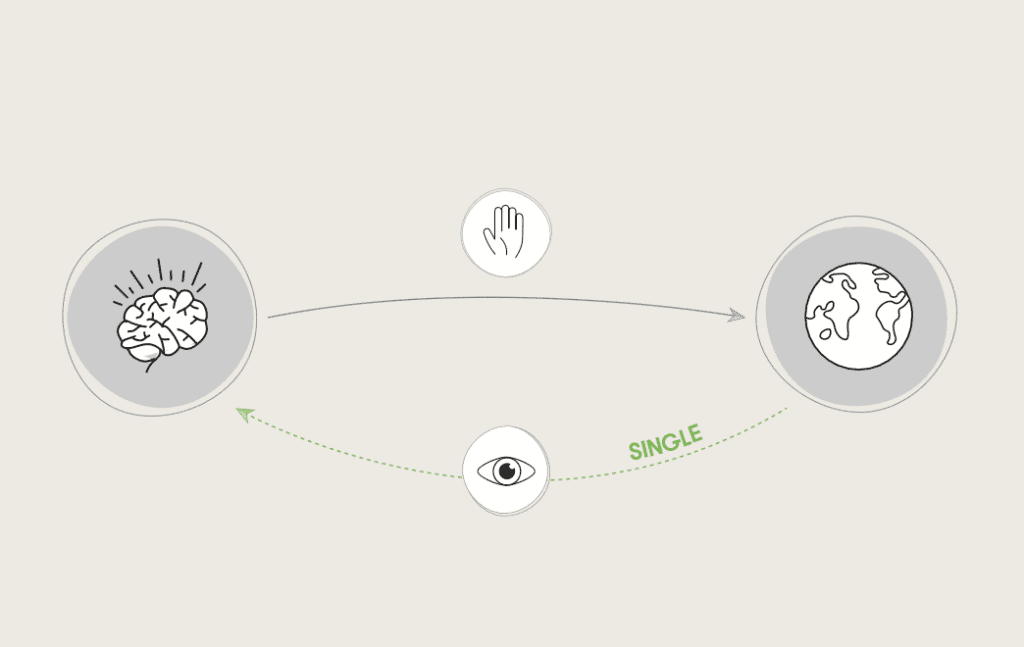
Single-loop learning is the most basic form of learning (even more basic than linear learning). We can observe it when a child learns how to walk or when learning to play an instrument. Learning, in this case, is a bodily (“sensorimotor”) interaction with the physical world.
To illustrate this, just think of a parent teaching their child to ride a bike without support. Rather than explaining the steps to the child, it’s about the physical interaction between parent and child.
It is way harder to conceive of the role a knowledge cloud can play in this. And this is also where things start to get interesting. While there could be embodied interaction with the knowledge cloud, nothing has been said yet about how such interaction might occur.
In the context of higher education, single-loop learning is evidently relevant for the (analog) arts or sports and areas of medicine that require bodily skill. But it is also relevant for teaching “purely cognitive” skills. Just think of the teacher’s physical act of calculating on a blackboard. There is an interactive back and forth between teacher and student that lasts until the process has finally become sufficiently stable to be carried out autonomously by the student.
Single-loop learning is a process that we usually accept implicitly as a means to teach and learn. Nonetheless, it seems that a knowledge cloud has not been thought of in the context of this embodied means of learning.
For single-loop learning to succeed, physical spaces need to meet complex demands. They not only need support the required physical interaction. Single-loop learning is also a socially sensitive process. Other people play a supporting, encouraging role, or their presence can also discourage the learner. (Just think of the demonstration effect.)
Single-loop learning might be a great opportunity to create intimate cyber-physical learning spaces in which smart tools enable physical action. However, single-loop learning is a highly embodied and socially interactive process that cannot be completely shifted into the virtual world. It is therefore critical that we design physical spaces for digitally augmented single-loop learning.
Double Loop Learning
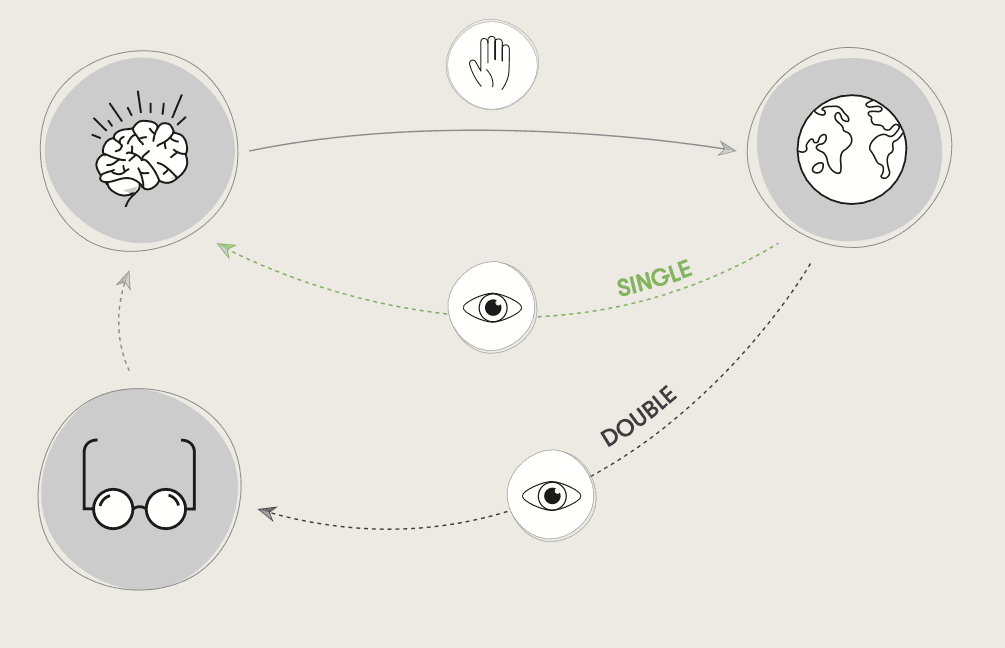
Double-loop learning assumes that knowledge is embedded in a frame of reference. For instance, when learning how to play an instrument or to perform a surgery, the student usually does not question how one does it. Usually, when being taught, she takes for granted that this is the “right” way of doing it. D
ouble-loop learning is about challenging such taken-for-granted assumptions. We form assumptions based on our interactions with the environment, through cultural learning processes, and education, etc. (In the case described above, the assumption might be that an experienced teacher knows the drill and is not to be questioned.)
In most cases, assumptions are not explicit. They determine how we learn without us being aware of it. However, we can change our implicit assumptions if we are able to open ourselves up to reflection and questioning. This often involves opening up to (highly trusted) peers so as to mutually explore one’s hidden assumptions.
Double Loop Learning aims to not only make assumptions explicit but also to systematically change them. This creates a new space of meaning in which already known phenomena can be seen from a new perspective.
Returning to our example from above, the interaction between parent and child: A child goes through the process of double-loop learning when it questions the assumptions on which first-order learning processes are based to begin with. An example of this would be when a child learns that parents do not do everything right and are fallible beings – in other words, when children question the authority of their parents and thus gain a completely new view on the world.
At first sight, the knowledge cloud seems to lack the capacity to facilitate double-loop learning. It is indeed hard to conceive of how interacting with the knowledge cloudcould help us challenge our assumptions. After all, it only operates on and stores explicable information. Yet, the knowledge we create or change through double-loop learning is highly implicit. (Moreover, even if the cloud might be able to make us aware of hidden assumptions and biases, there are ethical concerns about whether it should have such an ability.)
Hence, the knowledge cloud makes it almost inevitable that we train students in their capacity to question their implicit assumptions. After all, this will be an increasingly important skill for using the knowledge cloud responsibly. Double-loop learning involves questioning the reliability of a source and questioning its frame of reference. And this will be an indispensable ability considering the radical availability of continuously growing and changing knowledge
What does this mean for our physical spaces? Physical learning spaces that support double-loop learning must be “socio-epistemological” spaces that meet highly intricate demands: they must enable the exploration of implicit assumptions. To achieve this is to interact with trusted peers in a trusted environment. The basis of trust, however, grows through our embodied interactions in physical spaces. It is built in small (oftentimes minor) meetings and encounters, which barely happen in the controlled environment of our virtual worlds. Once trust is established, one can support the openness needed for students to questions their implicit assumptions in an interactive manner.
Triple Loop Learning
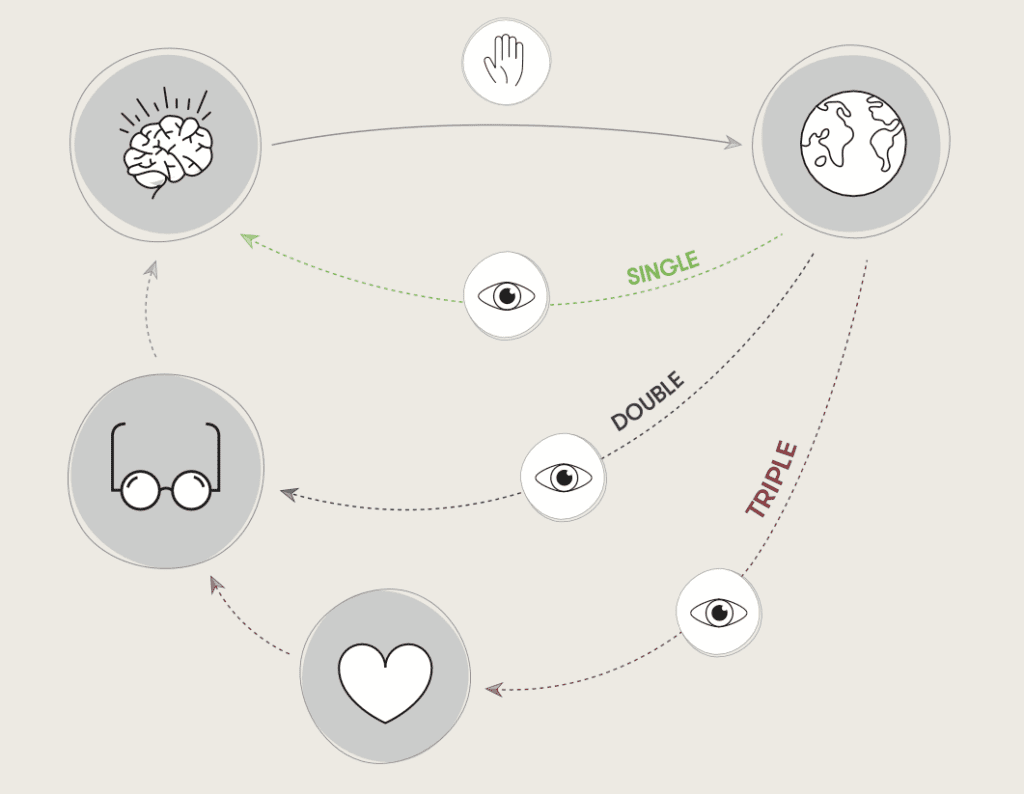
This takes us to the highest level of learning. While single and double-loop learning primarily aims at changes on the cognitive and affective level, “Triple Loop Learning”, according to Markus Peschl (2007), focuses on the “existential” level and thus on the person (and its unique identity). It aims at transforming who we are.
While for some, this might sound like an almost spiritual endeavor, it can also be considered a universities highest educational mandate. Indeed, modern-day universities are often criticized for “training” (“ausbilden”) students rather than educating them (“bilden”). Thereby, they often neglect the goals of the Humboldtian model of higher education demanding that universities must enable students to cultivate their character, doing so in an autonomous way.
Considering this, triple-loop learning is not primarily about teaching new skills or knowledge, but about enabling personal change and transformation. Triple-loop learning is about values, attitudes, and the person’s profound “habitus”. It is the most difficult type of learning because it is highly personal and “about oneself”.
To show why this type of learning will become more important in the working world: Triple-loop learning is particularly important for leaders, because only those who know themselves well can develop themselves as leaders in the best possible way and, by remaining open to their potentials, also to the benefit of their employees and the organization.
Yet, triple-loop learning might be considered an endangered species. In an academic world in which speed and productivity are mandatory, personal transformation might not be our first priority. Triple loop learning is a form of cognitive immersion that goes against the grain of the above-mentioned “smart learning environments”.
Yet it is the latter that will be accelerated by the emergence of the knowledge cloud. Even more so, it will become crucial to create decelerated and recreational spaces for triple-loop learning that can co-exist with the fast-paced digitized environment of the knowledge cloud. Hence, to create the cyber-physical spaces of the future, to create future learning landscapes, it becomes even more important to create physical refuges in which we can escape the fast pace of our world so that we can devote ourselves entirely to us.
What Does This Mean for Learning Spaces?
To conclude, we are observing the trend that future learning spaces are conceived of as cyber-physical spaces endowed with smart devices for teaching and learning. Yet, as of now, only the digital endowment of such spaces has been of interest. Physical spaces are treated merely as an appendix to be shaped without major constraints.
Yet, by looking at the ways we learn one can see that we must not disregard the physical and built environment. A significant part of our abilities to learn is based on physical embodied interactions, often in the presence of other students and teachers. It is critical that we first understand how and where smart tools such as the knowledge cloud can support (or undermine) types of learning. Doing so will yield a plethora of different framework conditions for designing physical spaces that are genuinely balanced cyber-physical learning landscapes – spaces that meet the deepest embodied demands of the learner.
To also refer to our own experience: Our Enabling Spaces technology has always pursued the goal of understanding the core processes of an institution, an organization, a company, and designing its spaces to enable precisely these core processes. Given the challenges of future learning spaces (the built environment of libraries and universities), our toolkit is particularly well suited to design the physical spaces our learning abilities need to sustainably explore the potential of the digital. One reason for this is that our understanding of knowledge has always assumed that the origin of human knowledge is not objects (i.e., symbols and data) but human action.
Are you in the process of designing a learning space of the future?
We are a multidisciplinary team with over 20 years of experience creating enabling and learning spaces for organizations. Our clients value the authentic, purpose-driven, and co-creative approach we bring to their projects. With a background in cognitive science, we will help you to deeply understand people’s needs.
Then, we will partner with you in realizing a highly effective learning space. If you’re looking for a research and design partner, we invite you to a free introductory call, where we talk about your current challenges and vision. Just pick a date in the form below.
References
Clark, A. (2003). Natural-Born Cyborgs: Minds, Technologies, and the Future of Human Intelligence (Issue 450). Oxford University Press.
Freigang, S., Schlenker, L., & Köhler, T. (2018). A conceptual framework for designing smart learning environments. Smart Learning Environments, 5(1), 27. https://doi.org/10.1186/s40561-018-0076-8
Friedman, C. P., Donaldson, K. M., & Vantsevich, A. V. (2016). Educating medical students in the era of ubiquitous information. Medical Teacher, 38(5), 504–509. https://doi.org/10.3109/0142159X.2016.1150990
Gambo, Y., & Shakir, M. Z. (2021). Review on self-regulated learning in smart learning environment. Smart Learning Environments, 8(1), 12. https://doi.org/10.1186/s40561-021-00157-8
Gros, B. (2016). The design of smart educational environments. Smart Learning Environments, 3(1), 15. https://doi.org/10.1186/s40561-016-0039-x
Haugeland, J. (1993). Mind embodied and embedded. In Y.-H. H. Houng & J. Ho (Eds.), Mind and Cognition: 1993 International Symposium. Academica Sinica.
Noh, Y. (2015). Imagining Library 4.0: Creating a Model for Future Libraries. The Journal of Academic Librarianship, 41(6), 786–797. https://doi.org/10.1016/j.acalib.2015.08.020
Peschl, M. F. (2007). Triple-Loop Learning as Foundation for Profound Change, Individual Cultivation, and Radical Innovation: Construction Processes beyond Scientific and Rational Knowledge. Constructivist Foundations.
Image by Giammarco at Unsplash